Fill out the form to get more information about the Fullstack Academy bootcamp of your choice.
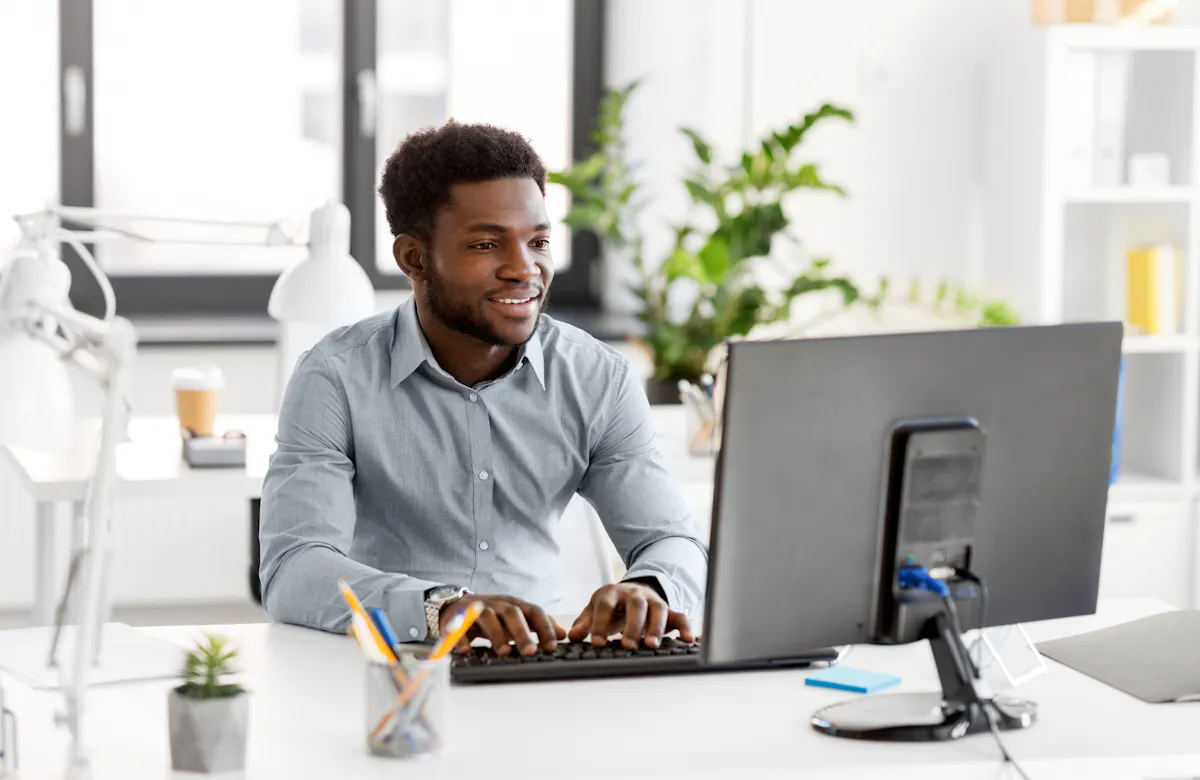
Addressing Bias in AI
Much attention has been paid to the potential for and the dangers of artificial intelligence (AI) tools such as ChatGPT and Bard. Some say that AI can be used to support fair and unbiased practices in certain fields, such as diversity, equity, and inclusion (DEI) efforts of human resources departments. But AI programs are just software, and all software is only as unbiased as its creators. AI can’t—yet—detect intentionally biased content, much less pick up on implicit bias. It can be a fantastic tool, but data professionals must be aware of and guard against potential bias in AI.
To learn more, check out the infographic below, created by Fullstack Academy’s Artificial Intelligence and Machine Learning Bootcamp program.

How AI Develops Bias
AI bias, also known as machine learning bias, refers to AI results that are prejudiced for or against certain groups. This occurs when the vast quantities of data used to train these automated systems contain biased information, reflecting historical inequity among humans. How does this happen?
Here are some of the ways AI systems can perpetuate human bias, however unintentionally:
- Misinterpreted data: drawing of faulty, incomplete, or unintended conclusions by algorithms
- Poor data: use of small or unrepresentative data sets
- Influenced data: introduction of erroneous information
- Excluded data: exclusion of data points that may have seemed unimportant at the surface level
- Biased data: use of data that preserves biases, stereotypes, or prejudices present in current systems
The bottom line reflects the old computer programming adage “garbage in, garbage out.” If the data used to train an AI system is flawed, the decisions that the system makes will be flawed. For example:
- An algorithm used by U.S. hospitals was supposed to predict which patients would need additional medical attention. But because it was trained with healthcare cost data alone—which lacks socioeconomic context—it falsely concluded that white patients needed more care than Black patients with the same symptoms simply because more money is typically spent on white patients.
- The Equal Employment Opportunity Commission sued language tutoring company iTutor Group in 2022, alleging that its recruitment software was intentionally programmed to reject older job applicants.
- Amazon trained its hiring system using data from 10 years of hiring decisions at the company. Because men dominate the information technology industry and Amazon’s hires, the AI system concluded that men made the best candidates, especially for tech positions already dominated by this demographic.
Effects of Bias in AI
Potential problems of bias in AI include discrimination against women, people of color, the working class, and those with disabilities. These systems may exacerbate unfair targeting or profiling by the police, underrepresent certain demographics in clinical drug trials, or overlook qualified candidates for hiring opportunities. Here’s a closer look at some consequences of biased AI results in three industries.
Criminal Justice
AI has the potential to heavily impact the criminal justice system due to its ability to process reams of data. Positive applications of AI’s use in criminal justice include monitoring closed-circuit TV (CCTV) for traffic accidents, scouring online activity for evidence of money laundering and human trafficking, analyzing forensic data, and comparing DNA results to help solve cold cases.
Negative outcomes include delivering poor facial recognition results for nonmale and nonwhite populations, increasing rates of pretrial incarceration for Black and low-income people, and increasing geographical discrimination due to AI-based surveillance leading to higher arrest rates in the areas being surveilled.
Healthcare
Machine learning and AI have the potential to transform patient care at a time when healthcare systems have a shortage of workers to serve an aging population. Positive applications of AI’s use in healthcare include detecting cancer in medical imaging that can’t be seen by the human eye, making presurgical planning more efficient, and helping overcome the shortage of pathologists.
Negative outcomes of AI in healthcare include reporting inaccurate oxygen saturation levels in the blood of Black patients versus their white counterparts due to faulty imaging, misdiagnosing skin cancer in nonwhite patients, and inaccurately diagnosing women’s symptoms as those predicting heart attacks.
Human Resources (HR)
If properly trained, AI could eliminate bias from hiring and help companies fulfill their DEI visions of more equitable workplaces. Positive applications of AI’s use in HR include screening resumes, allowing recruiters to spend more time with qualified candidates; answering candidates’ questions through chatbots; and eliminating unconscious human bias.
Negative outcomes include replicating past systemic inequities inherent in the data, analyzing candidates’ facial characteristics and drawing erroneous conclusions, and mischaracterizing assimilation techniques used by candidates with disabilities and other underrepresented groups.
How to Avoid Bias in AI
Given AI’s potential for improving hiring efficiency, health outcomes, and equitable justice, the existing potential for biases in AI needs to be eliminated, and steps must be taken to avoid introducing more. Here are some methods to exclude bias:
- Use training data that covers large, representative information.
- Check systems over time to preclude learned bias.
- Build consistent feedback and improvement procedures.
- Focus on transparency so everyone understands how the AI system reaches its decisions.
- Include checks and balances on humans to review their decisions and recommendations.
The U.S. Department of Commerce’s National Institute of Standards and Technology (NIST) supports a “sociotechnical” approach to reducing bias in AI. This approach is built on the understanding that technological solutions alone won’t work since AI must operate within a larger social context to be effective. The institute advises incorporating sociotechnical factors in the following ways:
Design
- Conduct sociotechnical analysis.
- Use social variations to provide context for data analysis.
Development
- Choose data sources with social factors in mind.
- Include impact assessments.
Deployment
- Launch an AI system only in the organization or environment for which it was designed.
- Include stakeholders.
- Explore cultural dynamics and norms.
- Monitor societal impacts.
Refining AI Systems to Reduce Bias
AI and machine learning have the potential to improve society in a wide number of ways. To harness AI’s potential and deliver better DEI results, those who are programming and training these systems must be aware of the possibilities for bias in AI and take steps to eliminate it. AI systems can only be as good as the data used to train them.
Sources
AIMultiple, “4 Ways AI Is Revolutionizing the Field of Surgery in 2023”
AIMultiple, “Top 3 Benefits and Use Cases of AI in Pathology in 2023”
AIMultiple, “Top 6 Radiology AI Use Cases in 2023”
CIO, “AI in Hiring Might Do More Harm Than Good”
Forbes, “The Problem With Biased AIs (and How to Make AI Better)”
Government Technology, “Justice-Focused Algorithms Need to Show Their Work, Experts Say”
Government Technology, “Risk Assessment Algorithms Can Unfairly Impact Court Decisions”
Indeed, “What AI Can Do for Your Recruitment—and What It Can’t”
Lever, “How to Reduce the Effects of AI Bias in Hiring”
NerdWallet, “AI Could Prevent Hiring Bias—Unless It Makes It Worse”
The New England Journal of Medicine, “Racial Bias in Pulse Oximetry Measurement”
Patterns, “Addressing Bias in Big Data and AI for Health Care: A Call for Open Science”
Recruiter.com, “Pros and Cons of Using AI in Recruiting”
Reuters, “Amazon Scraps Secret AI Recruiting Tool That Showed Bias Against Women”
Science, “Dissecting Racial Bias in an Algorithm Used to Manage the Health of Populations”
TechTarget, “What Is Machine Learning Bias (AI Bias)?”
Time, “We Need to Close the Gender Data Gap by Including Women in Our Algorithms”
U.S. Equal Employment Opportunity Commission, “EEOC Fiscal Year 2022 Agency Financial Report”