Fill out the form to get more information about the Fullstack Academy bootcamp of your choice.
05.13.2025
Should Coders Specialize or Generalize to Succeed in Their Career?
By The Fullstack Academy Team
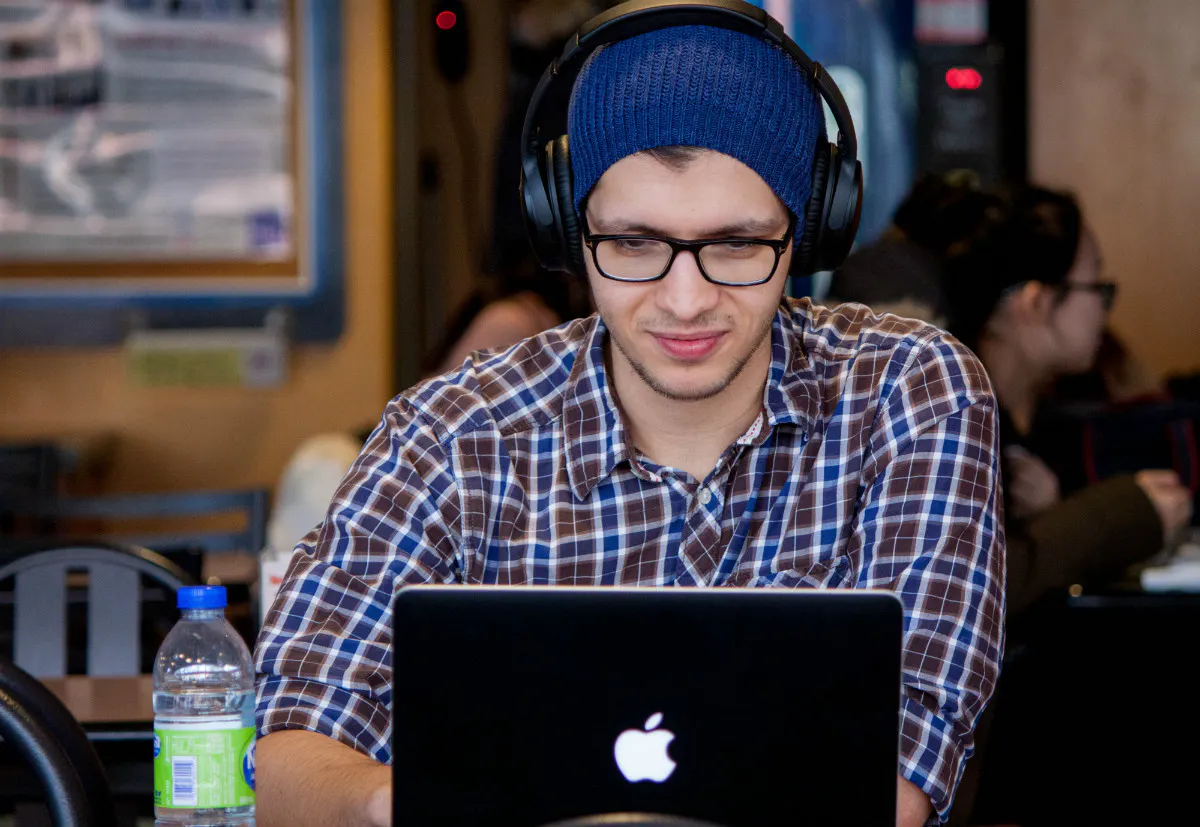
Last updated on June 2024
Many web developers face a choice: become an expert in one language, framework, or focus area (front-end, for example)), or broaden their skills to oversee development in multiple languages and technologies (full stack).
Some coders love a specific language, like JavaScript, and want to explore its full functional range (which, for JavaScript, is immense!). Others prefer to generalize their expertise.
To put it another way, all full stack developers are software engineers, but not all software engineers are full stack developers.
But what about new software engineers? Do you need to specialize right away, or should you learn as much as possible across different languages? This article explores the paths you can take to navigate your coding career!
The Case for Specialization
As we delve deeper into the "Is it better to specialize vs. generalize in coding?" debate, let's first explore the case for specialization. This approach involves becoming an expert in a specific programming language or development area. Here, we'll uncover the advantages of becoming an expert in a specific programming language or development area, while also acknowledging the potential drawbacks to consider.
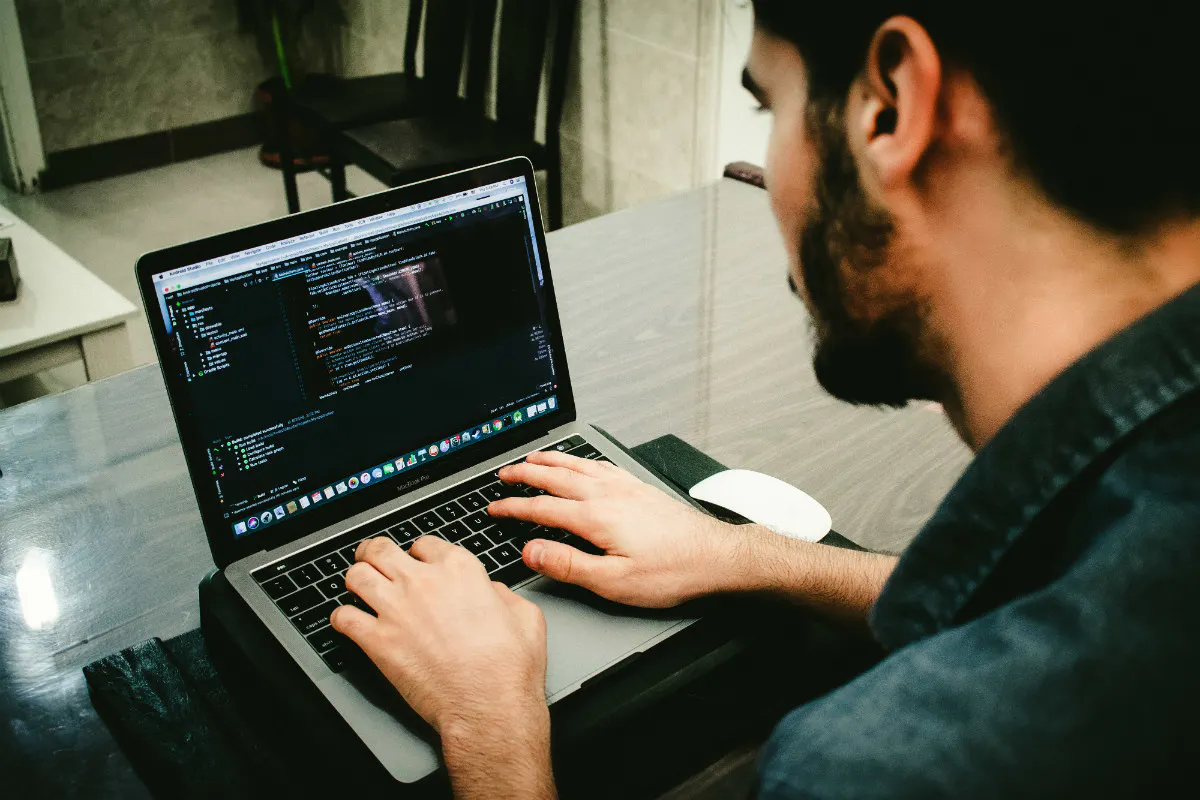
Ready to break into tech?
Learn the #1 programming language in 13 to 22 weeks with our live online coding bootcamp.
Benefits of Specialization:
Stand Out to Employers: Companies often seek developers who can serve as the organization’s go-to subject matter expert for a specific language like Python or Java. This deep knowledge makes you a valuable problem-solving resource, which employers love.
Learn Like a Pro: Specializing in one language first builds a strong foundation for learning additional languages and other coding concepts more effectively. It's like learning to ride a bike before jumping on a motorcycle—you'll know the basics of how to get started when it’s time to level up!!
Unleash Hidden Skills: Specializing can open your eyes to a whole new world within your chosen language. You might discover hidden gems in functionality you never knew existed—like automated testing and performance quick-fixes. This extra knowledge makes you a more well-rounded developer.
Keep Up with Tech's Speed: The tech world is moving faster every day! Specialization helps you focus on a specific technology to become an expert. This ensures you stay relevant in a fast-paced industry.
Sharpen Your Focus: Full stack development can be overwhelming. Specialization lets you hone your skills in a specific area, like front-end design. This can make you more efficient and less stressed when working on complex projects.
Drawbacks of Specialization:
Limited Job Opportunities: While specialization can make you highly desirable for specific roles, it can also limit your overall job prospects. Smaller startups or early-stage ventures often seek developers with a broader range of skills. Being hyper-specialized can then make it challenging to qualify for wide-ranging opportunities.
Adapting to Change: Tech is constantly evolving, with new languages and tools popping up all the time. Specializing in a technology that becomes outdated can leave you scrambling to catch up.
Tunnel Vision: Deep focus on a single area can lead to "tunnel vision," where you lose sight of the bigger picture. A well-rounded understanding of different programming paradigms and development processes can be beneficial, even within a specialized role.
Loss of Creativity: Focusing solely on technical expertise can stifle creativity. General skills, like understanding user experience (UX) principles, can help you approach problems from different angles and potentially lead to more innovative solutions.
Ready to break into tech?
Learn the #1 programming language in 13 to 22 weeks with our live online coding bootcamp.
The Case for Generalization
We've explored the advantages of specialization, but what are the advantages of generalization in coding professions?
This approach involves developing a broader skill set across multiple programming languages and development areas. While specialization offers deep expertise in one language, generalists gain a wider range of abilities in multiple languages or technologies. Let's delve into the benefits and drawbacks of this path.
Benefits of Generalization:
More Job Openings: Generalists have more skills, so more companies want to hire them!
Efficient Problem-Solving: Generalists are more knowledgeable in all components of a program’s inner workings. Usually, that translates to the ability to build and implement fixes more efficiently than specialists. Knowing many languages allows you to pick the right tool(s) for the job.
Future-Proof Your Coding Skills: Tech is always changing, with new languages popping up all the time. Developers often need to learn new languages on the fly. Being a generalist helps you adapt to these changes. Think of languages like tools in your coding toolbox: the more you have, the easier it is to tackle complex challenges.
Drawbacks of Generalization:
“Jack of All Trades, Master of None”: While generalists can do a lot, they might not be the absolute best at any one thing. Specialists who focus on one language or function can work within those parameters more efficiently.. It’s all about balance.
Missing Out on Super-Skills: Specialists can become coding experts in their chosen language. They might know all the tricks and secrets that generalists don't.
The Potential for More Work: Generalists have to keep up with more coding knowledge than specialists. It can take more time to stay updated on many languages compared to focusing on just one.
Choosing between specialization and generalization is a personal decision. The ideal path depends on various factors and personal preferences. In the next section, we'll explore strategies to help you make an informed decision in choosing between specialization and generalization for your coding journey.
Ready to break into tech?
Learn the #1 programming language in 13 to 22 weeks with our live online coding bootcamp.
How to Choose Between Specialization and Generalization
So, how do you decide between becoming a specialist or a generalist? Here's a roadmap to navigate this coding crossroads:
Consider Your Interests: What excites you most? Delving deep into any given subject, or exploring a broader range of associated subjects?
Think About Your Goals: Do you prefer to serve as the go-to expert of a specific function, or do you prefer to have a hand in various projects?
Leverage Your Strengths: Are you detail-oriented (specialist) or do you tend to get bogged down by details (generalist)?
Remember, there's no one-size-fits-all answer. The best path is the one that aligns with your interests, goals, learning style, and strengths. You can even experiment with both approaches and see what clicks!
Still Not Sure? Become a T-Shaped Developer Instead
Both sides of the debate present good points. On the one hand, we’ve seen arguments that tech changes too quickly to generalize, and that you should become an expert in one language before learning others. And on the other hand, there are people who feel learning multiple languages keeps you upwardly mobile and open to opportunity.
Specialization is important, but there are few specialists who weren’t first generalists. That’s because it’s usually impossible to become a good specialist without first building out a broad base of programming knowledge. This progression is referred to as “T-shaped” learning: Students first become proficient in a wide range of languages, before specializing in a single area of expertise.
At many bootcamps, the approach is often a combination of all the above—in what might be called an “upside-down T.” That’s the idea that when you’re transitioning from one career to another, as many bootcamp students are. Trying to learn eight different languages at once is going to be overwhelming and won’t prepare you to get a more specialized coding role.
Since there is no one preeminent tech stack, —learning any single stack all the way through can help you understand not only what it takes to learn a whole new language, but also how you learn best.
Once you’ve figured out your own styles, your own strengths and weaknesses, and how one set of technologies fits together to work as a whole, you’ll have everything you need to learn another programming language, or focus area within that language, at a later date.
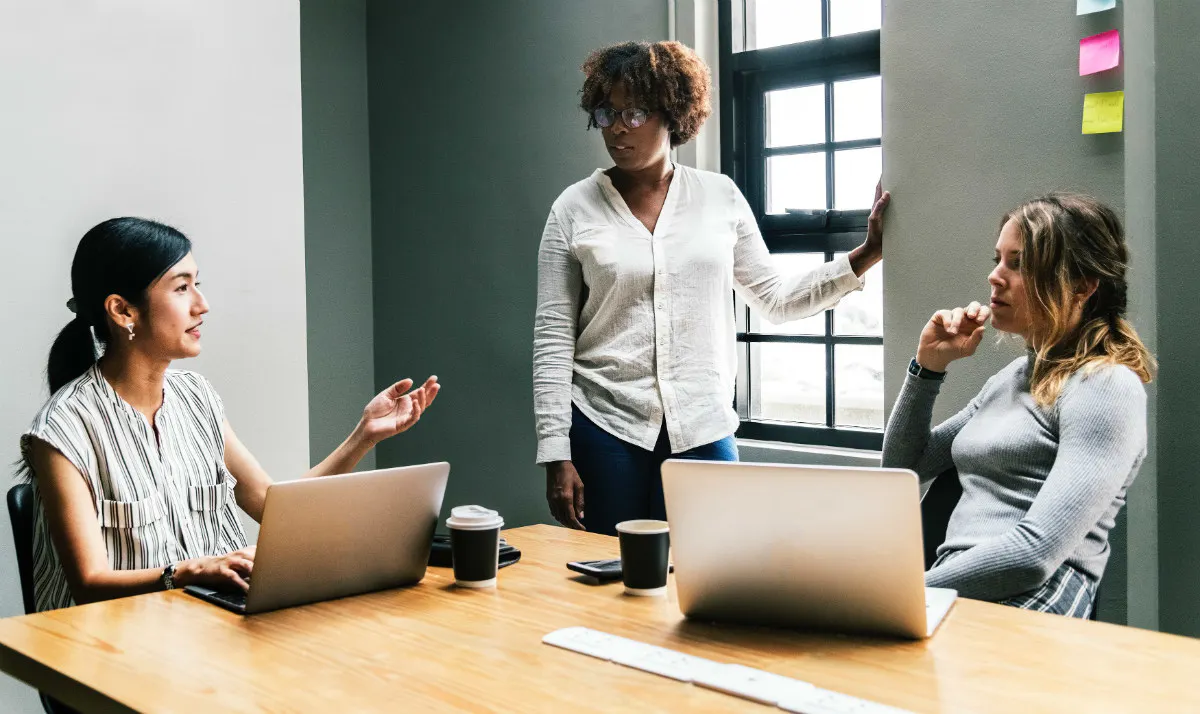
And Don’t Forget About Soft Skills
Because coding is so tech-focused, there is a tendency for developers to put their technical skills before everything else. However, “soft” skills (interpersonal skills) like emotional intelligence and conflict resolution, are crucial for long-term success in any position, and especially important if you ever want to progress into a more senior or managerial role.
Ready to break into tech?
Learn the #1 programming language in 13 to 22 weeks with our live online coding bootcamp.
Start With JavaScript
Whether you choose to generalize, specialize, or something in between, you have to start somewhere. Begin by identifying what language you will learn first. Right now, there are few better languages to learn than JavaScript.
JavaScript continues to be one of the top programming languages, and over 60%of professional developers in the Stack Overflow study agree that they have used JavaScript. Whatever direction programming takes you—whether you specialize forever, generalize forever, start narrow and broaden, start broad and get more specific over time—the most important thing is just to get started. You’ll figure it out from there.
FAQs
1. What is the difference between specialization and generalization?
Specialization is focusing on a specific coding area or language to become an expert. Generalization is learning a broader range of coding skills to be adaptable across different projects.
2. What is generalization and specialization in C++?
In C++ specifically, generalization involves creating base classes with common functionalities. Specialization allows you to create subclasses inheriting these functionalities while adding specific details.
3. Is specialization better than generalization?
There's no single "better" option. Specialization makes you a deep expert, while generalization offers more job options. Choose based on your interests, learning style, and career goals.
Ready to dive into JavaScript today? Start learning the basics with our 'Intro to Coding' free course, or learn more about the Fullstack Academy Coding Bootcamp.